Predicting the Development of Alzheimer’s Disease with Artificial Intelligence
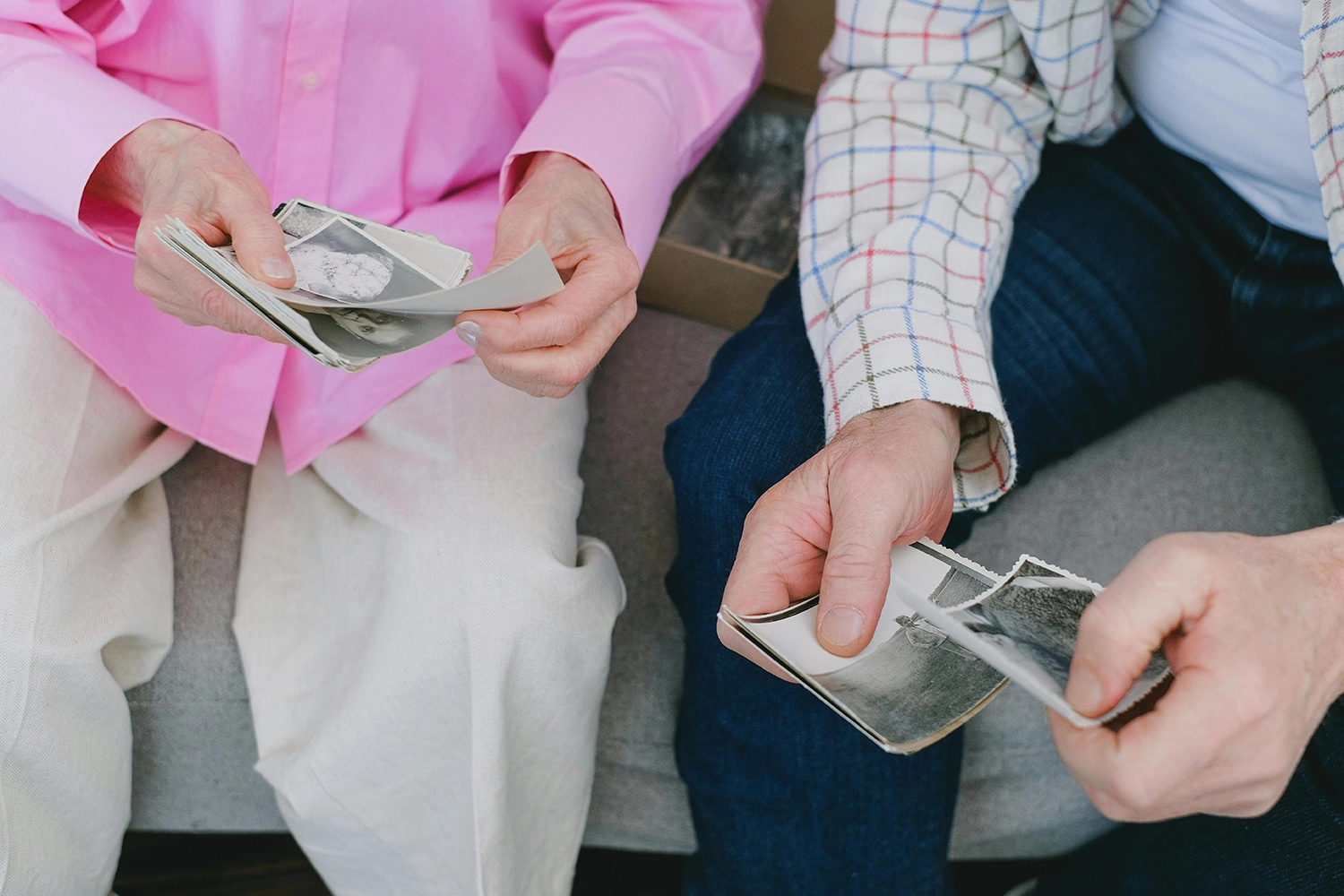
PREMIUM CONTENT for MEMBERS ONLY
In a previous post, I discussed a study by Jinzhuo Wang and a research team from Peking University, which used AI tools that can predict someone’s biological age by analyzing biological details obtained from different parts of the body, so called biomarkers. In their study, which was published in the journal Proceedings of the National Academy of Sciences (PNAS) they used the appearance of the brain in MRI scans, the health of the eyes, in particular the retinal blood vessels, and even the visual appearance of the face and the tongue. They hypothesized that the modelling of the impact of chronic diseases, such as cardiovascular disease, chronic kidney disease, diabetes, hypertension, and stroke will require integrated information from multiple systems. What is most exciting about this approach is the fact that it may not only be applicable to efforts to estimate biological age, but also to all attempts to better understand complex chronic diseases and to develop curative treatments for them.
“The aim of the study was to identify several early risk clinical factors to predict a person’s risk to develop Alzheimer’s disease (AD) up to seven years before any symptoms occur.”
In a recent study, published by Alice Tang and a team of investigators from the University of California in San Francisco (UCSF) in the journal Nature Aging, the investigators used a different AI approach to analyze a vast amount of Electronic Health Records (EHR). The aim of the study was to identify several early risk clinical factors to predict a person’s risk to develop Alzheimer’s disease (AD) up to seven years before any symptoms occur. If successful, this revolutionary approach would enable physicians to apply therapeutic interventions early enough to delay or even prevent the full blown disease.
“In the absence of new curative treatments, efforts to deal with these diseases have focused on finding ways to help people at risk before their condition gets worse and can’t be reversed.”
Neurodegenerative diseases including AD and Parkinson’s disease (PD; see also the companion post by Madelaine Leitman in this blog) are a group of serious chronic conditions that are becoming more common as populations age and as environmental and lifestyle factors, including dietary habits have changed dramatically. AD is the most frequent type of dementia in people over 65, causing memory loss and other mental problems that are a huge burden on patients and caregivers and on the medical system. In the absence of new curative treatments, efforts to deal with these diseases have focused on finding ways to help people at risk before their condition gets worse and can’t be reversed. Thanks to new developments in research, including better ways to detect AD and new treatments that can slow the disease down, there has been progress in identifying and managing AD, although the factors that cause AD remain poorly understood. These limitations are largely due to the fact that there is insufficient long-term data, or data that connects the disease’s molecular makeup with its clinical symptoms. However, ongoing research efforts by large international consortia (like the Alzheimer’s Disease Metabolomics Consortium (ADMC) having access to a vast amount of biological, molecular, and genetic data from research centers around the world and the analysis of large databases using AI is the most promising development in unraveling the complexity of this disease and in developing effective treatments.
“One source of large data sets for clinical research are EHRs, the comprehensive clinical health records generated with each patient visit…”
One source of large data sets for clinical research are EHRs, the comprehensive clinical health records generated with each patient visit at health centers across the US. These vast data sets have, in recent years, become a valuable source of ongoing information that can help us understand and predict complex diseases like AD. They’ve been used to deeply analyze AD characteristics, find related risk factors and theories, and build models that can forecast dementia from health records. These records can capture a doctor’s current understanding of a patient’s health history, which can be used to make affordable, early predictions in primary care settings. However, while machine learning (a type of AI) has been applied to these records to predict dementia, there have been challenges, such as the models not being specific enough for AD, lacking biological context, or depending on data that isn’t always available.
“By combining decades of research across different biological areas, it has become possible to understand the complex relationships between genes, diseases, and treatments.”
With the growth of data science and the collection of diverse biological data, there’s a growing interest in using sophisticated methods to gain insights into complex diseases. By combining decades of research across different biological areas, it has become possible to understand the complex relationships between genes, diseases, and treatments. The UCSF investigators’ approach tries to connect various types of data to shed light on the potential underlying causes of AD and how they relate to what we see in patients. In the current study, Tang and colleagues explored how to use vast amounts of EHR data to predict the risk of developing AD.
“The investigators trained statistical models derived from EHR data, …and predicted AD onset …with 72% accuracy.”
In their study, the investigators used EHRs data from UCSF Medical Center to build models that predict who might develop AD several years later, to explore the biological connections between key risk factors and the disease, and to identify differences between female and male patients. They made sure that their models considered differences in demographics and other potential biases and used a diverse set of biological data to explain their findings and checked their predictions against other data sets and genetic analyses. The investigators trained statistical models derived from EHR data, so called random forest models, and predicted AD onset on a cohort of 749 individuals with AD and 250,545 controls with 72% accuracy.
By using AI and the clinical database, the researchers identified several early risk factors for AD seen in both men and women, including high blood pressure, high cholesterol, and Vitamin D deficiency. In addition to these early risk factors identified in both men and women, researchers also discovered a few sex-specific risk factors, including erectile dysfunction and an enlarged prostate in men, and osteoporosis in women.
Even though the results are not completely novel, these findings using an exciting new approach to characterize risk factors for a complex disease, they add to other research concerning these three medical conditions and their possible link to an increased risk for AD or dementia. However, several limitations have to be kept in mind: The study findings are correlational and do not increase our understanding of factors that play a causal role in AD. The findings are population-based and may not apply to all individuals with these risk factors.
“Many lifestyle factors … including psychological stress, social isolation, dietary habits, regular physical exercise and emotional factors like happiness are not captured in the HER.”
Lifestyle factors which are likely to play a role in increasing or mitigating the risk for AD, including psychological stress, social isolation, dietary habits, regular physical exercise, and emotional factors like happiness are not captured in the HER and did not enter into the AI generated prediction. In view of these limitations and until science will reveal specific causal mechanism underlying the development of AD, it may be good to following the lead author Alice Tang’s recommendations of how to reduce the risk of AD in genetically vulnerable individuals:
“Nevertheless, a doctor can take the population level risks to counsel patients to aim to control their cholesterol, engage in exercise, take plenty of calcium/vitamin D, or treat osteoporosis to minimize the influence of those diseases on the risks…Ultimately, in the future, we imagine a personalized model in the clinic will be able to not only predict risk but also list the risk factors for the individual patient in front of the doctor for more targeted advice and treatments.”
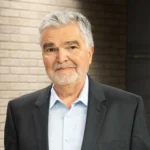
Emeran Mayer, MD is a Distinguished Research Professor in the Departments of Medicine, Physiology and Psychiatry at the David Geffen School of Medicine at UCLA, the Executive Director of the G. Oppenheimer Center for Neurobiology of Stress and Resilience and the Founding Director of the Goodman-Luskin Microbiome Center at UCLA.