A Revolutionary Way to Estimate Biological Age and Predict Health Outcomes

PREMIUM CONTENT for MEMBERS ONLY
Aging refers to the progressive decline, in an organism’s ability to meet physiological demands. This decline is highly variable, both in terms of how it affects different individuals at different ages, and how it affects different biological functions, ranging from cognitive decline to loss of muscle mass. A good example for this variability is cognitive function: while a person may live into their hundreds without noticeable decline, an increasing number of people are affected by cognitive decline starting in their 60s.
Biological age transcends the simple count of years since birth. Unlike the straightforwardness of chronological age, biological age reflects our body’s condition—a cumulation of environmental impacts, lifestyle choices, and genetic predispositions. It’s an aggregate marker of how our cells, tissues, and organs are faring against the sands of time and the stressors of life.
“… physicians could better identify people who might be at risk of getting age-related diseases early on, which would help to prevent and treat these conditions sooner.”
Focusing on biological age is a way of measuring age that looks at the physical and functional changes in our bodies. By figuring out someone’s biological age, physicians could better identify people who might be at risk of getting age-related diseases early on, which would help to prevent and treat these conditions sooner.
Understanding biological age is paramount because it’s more than a number—it’s a narrative of our health that can guide us to make informed lifestyle modifications. As Michael Snyder, a distinguished geneticist at Stanford, points out, knowing where we stand biologically can empower us to take charge of our health trajectory.
“…quest for a holistic assessment of biological age has become more urgent.”
The quest to quantify biological age has seen various incarnations. Initially, researchers sought answers in DNA methylation patterns—molecular signposts indicating which genes are dialed up or down as we age. This pursuit expanded to assessing metabolic markers and inflammatory proteins commonly checked in annual health screenings. Yet, as our understanding of aging’s complexity has grown, the quest for a holistic assessment of biological age has become more urgent. Enter the transformative potential of AI. In an innovative leap, scientists are now able to deploy AI to sift through vast number of 3D images of the face, analyze brain scans, and probe protein levels in the blood, weaving together a detailed tapestry of our biological age.
Biological age is a biomarker of chronological aging and has been used to predict the development of certain age-related chronic diseases, Alzheimer’s disease or cardiovascular disorders. Understanding and predicting how our bodies age has been challenging for scientists, healthcare providers and patients. The traditional way of using chronological age in combination with certain risk factors (incl. cholesterol, arterial blood pressure, BMI, lifestyle and diet), is not precise enough as it doesn’t consider the different rates at which our bodies age or the various health challenges we might face.
“…utilization of biological age to its full potential will undoubtedly need to consider the heterogeneous nature of aging.”
AI approaches have been developed to predict biological age from a number of biomarkers of aging, such as leukocyte telomere length, DNA methylation–based epigenetic clock, brain image–derived brain age, retinal age, and facial age. The retina in particular has been recognized as a “window to the brain” due to the presence of central nervous system–derived fibers in the optic nerve, as well as similarities in the expression of several inflammatory and immune modulators. The retinal age gap, the difference in the predicted retinal age and the chronological age, has been used to assess brain health. Facial age has also emerged as a potential predictor for skin health. For example, the investigators hypothesized that tongue images may be a potential indicator for oral and gut microbiome exposure, and may reflect the state of oral and gastrointestinal health emphasizing the prominent role of the brain gut microbiome system in overall health. Interestingly, the detailed assessment and description of the tongue has been an essential part of Traditional Chinese Medicine for thousands of years. However, while estimation of the biological age of specific organs or systems may be useful to derive information regarding organ-specific diseases, utilization of biological age to its full potential needs to consider the heterogeneous nature of aging.
To overcome the limitation of conventional assessment of disease risk and individual biomarkers, Jinzhuo Wang and a research team from Peking University, China have been creating AI tools that can predict someone’s biological age by analyzing biological details obtained from different parts of the body, so called biomarkers. In their study, which has recently been published in the journal Proceedings of the National Academy of Sciences (PNAS) they used the appearance of the brain in MRI scans, the health of the eyes, in particular the retinal blood vessels, and even the visual appearance of the face. They hypothesized that the modelling of the impact of chronic diseases, such as cardiovascular disease, chronic kidney disease, diabetes, hypertension, and stroke will require integrated information from multiple systems.
The PNAS study suggests that we need to consider all the different ways our bodies age together. The researchers used a special AI model that looks at thousands of images of different parts of the body to predict biological age. Their model was trained on a diverse dataset, including images from the face, tongue, and retina. It unearthed patterns and indicators that elude the human eye, like the significance of the tongue’s center, the areas surrounding the eyes, and the retinal spots dense with blood vessels—all reflections of our aging process.
“The investigators trained their model using facial, tongue, and retinal images from 11,223 healthy subjects…”
The investigators trained their model using facial, tongue, and retinal images from 11,223 healthy subjects and demonstrated that using a fusion of the three image modalities achieved the most accurate biological age predictions. Participants were observed over five years, with their health markers meticulously recorded. They validated their approach on a test population of 2,840 individuals with six chronic diseases and obtained significant difference between chronological age and biological age than that of healthy subjects.
Deep learning technology, particularly the Transformer model used by Wang and his team, originally fine-tuned for language processing tasks like ChatGPT, has now been adapted for image analysis. This has enabled a radical enhancement in computer vision, crucial for interpreting the subtle cues of aging in medical images.
This trifold approach stands in stark contrast to singular measurement methods. It accounts for environmental influences visible as wrinkles on our face, neural and vascular health mirrored in the retina, and gastrointestinal well-being and microbiome health indicated by the tongue’s appearance.
“The AI model’s predictions of biological age…were strikingly accurate.”
The AI derived biological age was generally higher in people with diseases or bad health habits, making it a strong way to predict the risk of chronic diseases. The investigators showed that this difference has the potential to be utilized as a standalone biomarker or in combination with other known factors for risk stratification and progression prediction of chronic diseases.
The AI model’s predictions of biological age in healthy individuals, acting as a proxy for health in the absence of chronic conditions, were strikingly accurate. The study also delved into unhealthy subjects, noting an increased biological age in those with chronic conditions or detrimental habits. The age differences (AgeDiff), a term coined for the disparity between biological and chronological age, revealed a greater predisposition to age-related diseases as it increased.
The implications of these findings are profound. The AgeDiff metric outperformed traditional health indicators in predicting the onset of diseases. When paired with other health metrics, it provided an even more precise forecast, suggesting a revolutionary approach to preventative healthcare.
Looking forward, the research team is honing their model, incorporating a broader range of variables, and expanding their studies across different ethnicities. They aim to leverage AgeDiff not only to predict but to proactively combat the emergence of chronic diseases by personalizing interventions.
“The ultimate goal of this endeavor is to democratize medicine.”
The team is working on user-friendly tools, such as smartphone attachments and apps, that could allow individuals to assess their biological age easily without expensive diagnostic tests. Such a tool could simplify and make health monitoring more accessible, minimizing the need for invasive tests and possibly reducing healthcare costs.
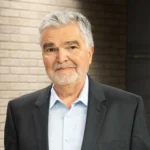
Emeran Mayer, MD is a Distinguished Research Professor in the Departments of Medicine, Physiology and Psychiatry at the David Geffen School of Medicine at UCLA, the Executive Director of the G. Oppenheimer Center for Neurobiology of Stress and Resilience and the Founding Director of the Goodman-Luskin Microbiome Center at UCLA.